EVALUATION OF AESTHETICITY OF WOMEN'S DRESS MODELS BASED ON DEEP LEARNING MODELS
DOI:
https://doi.org/10.61151/stjniet.v9i4.661Ключевые слова:
machine learning, aesthetics assessment, women's dresses, expert assessment, neural network modelsАннотация
Currently, the assessment of aesthetic appeal is conducted through a visual analysis of the product, which includes a professional evaluation of its appearance in terms of harmony, proportionality, and originality. These assessments are based on the subjective perception of experts in the fields of fashion or design. This study presents the results of an aesthetic evaluation of women's dress models using deep learning neural network algorithms. To prepare the database of dress images used for training the network to assess aesthetic appeal, the following criteria were selected as key parameters of aesthetics: novelty of the model and construction, and the degree of compositional refinement of the design. For building the neural network model and conducting computations, the “Google Colaboratory” cloud platform was utilized, as it is specifically designed for implementing machine learning and deep neural network algorithms. Based on the proposed neural network architecture, a program was developed for recognizing the aesthetic appeal of images.
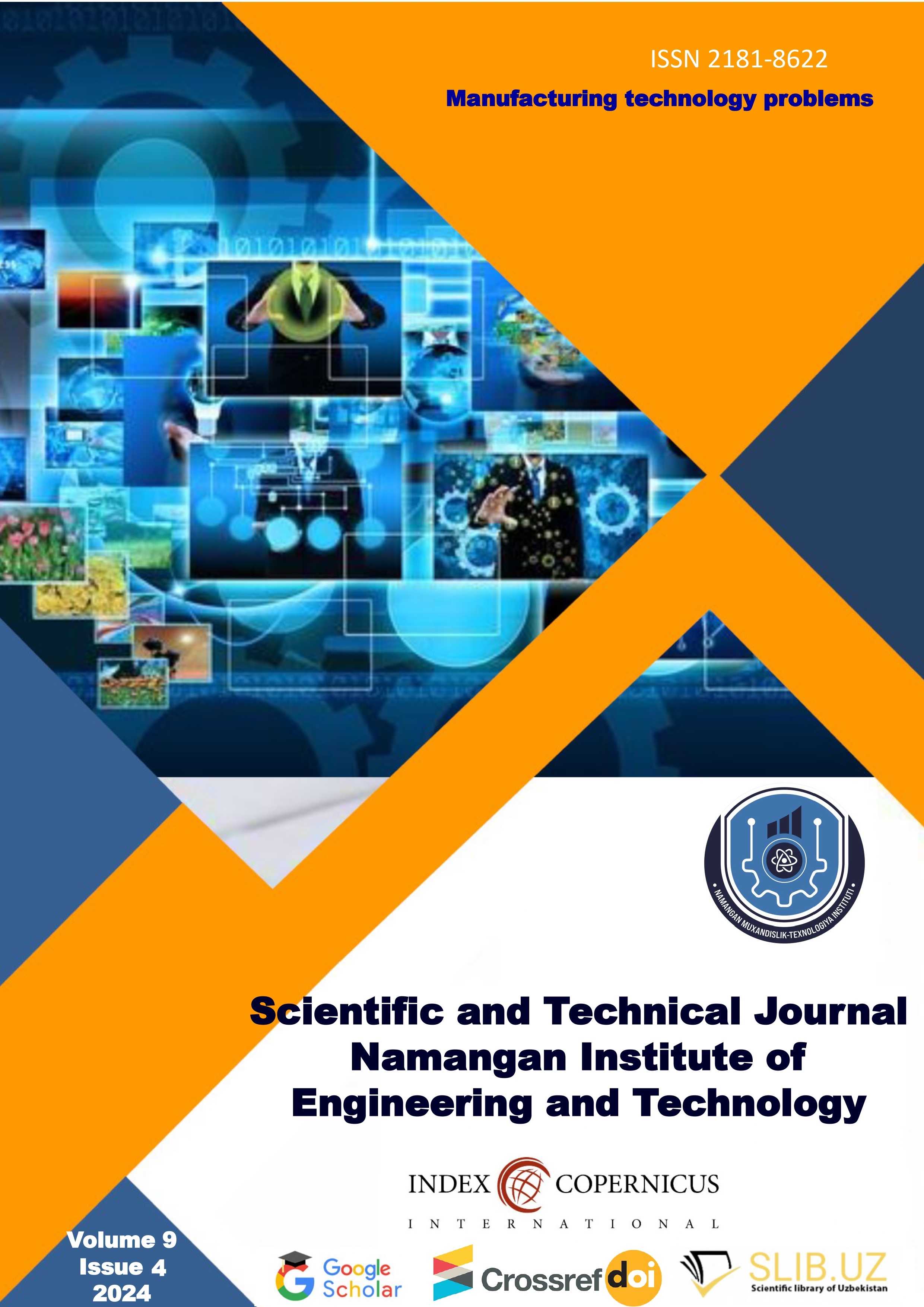